Issue 129
Term 2 2024
Can a chatbot write like an Aussie?
In a research project supported by a Reading Australia Fellowship, Bridget Forster investigates how generative AI impacts the Australian literary voice, exploring strategies to educate students about AI's cultural biases and limitations.
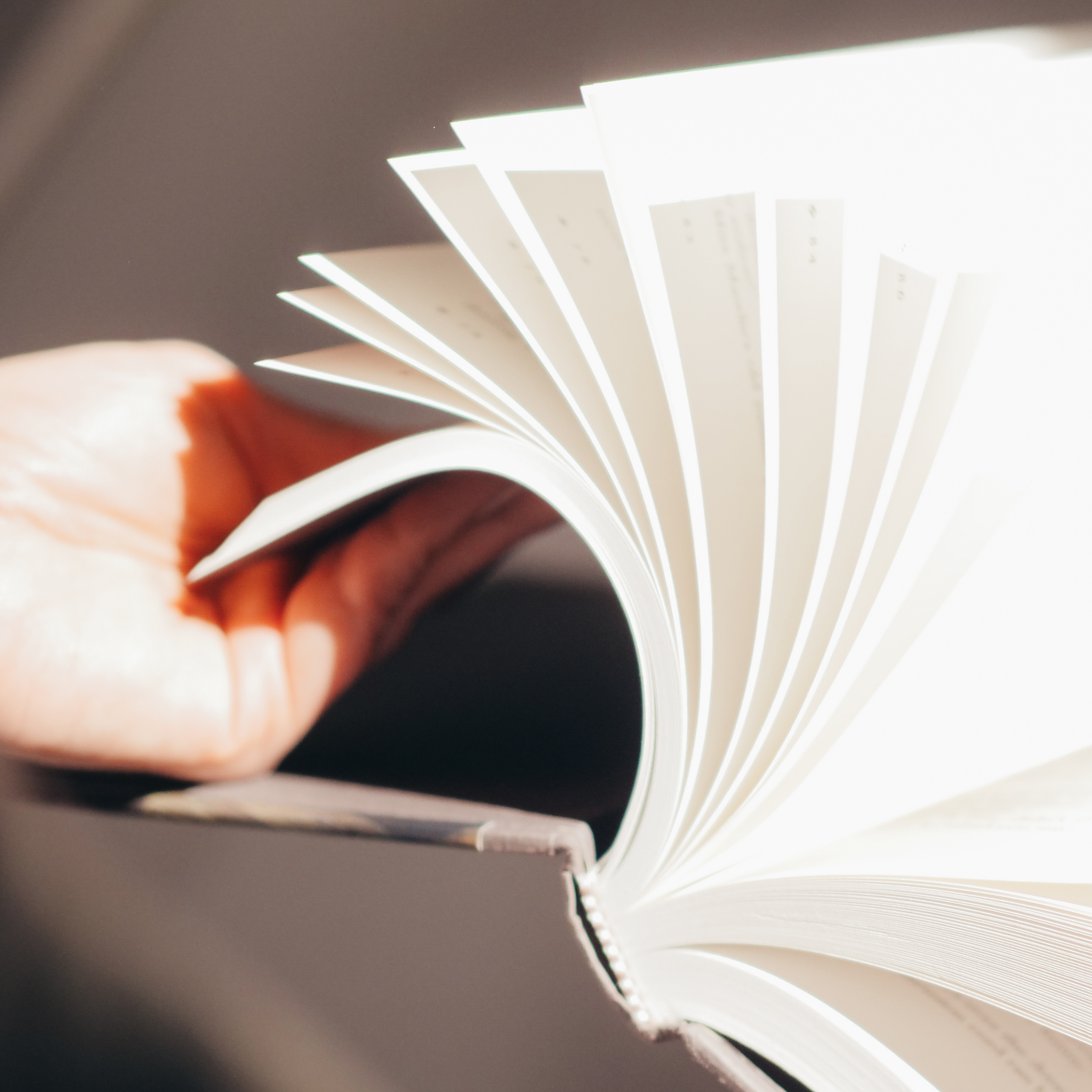
Can a chatbot write like an Aussie? Over the past few months, supported by a Reading Australia Fellowship, I have started researching how generative AI might be used to interrogate the notion of an Australian literary voice. Through my inquiry, I have gained not only a greater appreciation of the computational might of these tools, but also a clear sense of how we might make their limitations and cultural bias apparent to students.
What is the Australian Voice?
Although there is a multiplicity of Australian voices, my discussions with Australian YA authors revealed a few common threads.
There is a certain informality in the way Australians speak and write. This may be apparent in a looser sentence structure, and our preference for abbreviation, informal language Can a chatbot write like an Aussie? and vernacular. According to one author, Australians have a directness that contrasts with an American earnestness and fear of offending. There is perhaps also greater tolerance for flawed characters, who lie and transgress. Our unique landscape looms large in many Australian works, offering a clear sense of place to the point where the landscape is almost a character itself. Then there are the references to food, which often reflect our rich migrant heritage, as well as references to music and outdoor lifestyle, all of which make our Australian stories distinct. Importantly, First Nations’ knowledge and culture are becoming increasingly prominent in Australian YA, as greater numbers of works authored by Aboriginal and Torres Strait Islander writers are published.
With the advent of ChatGPT, AIgenerated fiction threatens to flood the literary market with low-quality, derivative work. In February 2023, the editors of three science fiction magazines reported being overwhelmed by short stories authored by chatbots, forcing them to pause and refuse submissions (Levenson, 2023). At the local level, content generated by large language models (LLMs) has seeped into most facets of Australian communications, whether it be the humble school newsletter, real estate ad, corporate email or parliamentary speech (Hepworth, 2023). The profusion of chatbot-authored texts threatens to dilute our distinctive Australian voice with a bland, culturally homogenous alternative that is compromised by embedded values regarding gender and cultural identity that are far from the national ideal of ‘a fair go’.
The Australian Framework for Generative AI in Schools stipulates that students should learn about how LLMs work and their limitations. It is through the prism of Australian voice in youth literature that many of these biases and limitations can be made plain for students. By using AI-generated texts as a foil against authentic Australian literature, students can come to appreciate the nuance of stories created in their own locale. This involves the teacher librarian selecting passages from Australian YA novels and using a series of prompts prescribing equivalent settings, characters and scenarios to create an AI-generated text that has a similar length and premise. Upon comparison, students might uncover the following limitations of LLMs.
LLMs present an outsider view of Australian culture
The datasets that power LLMs often contain American biases and stereotypes, despite their broader use internationally (Caliskan, 2021). This is due to a combination of inputs and filtering. Dodge et al. found that over 50% of websites in the Colossal Cleaned Crawled Corpus (C4-English) – a primary dataset for training many LLMs – were hosted in the USA. Websites hosted in the next four most populous English-speaking countries – India, Pakistan, Nigeria and the Philippines – consisted of a mere 3.6% of the dataset collectively. Furthermore, when datasets are filtered, the process disproportionately removes text about people from minority backgrounds (Dodge et al., 2021). These factors contribute to the monocultural nature of LLMs. In fact, when Jill Walker Rettburg, a Professor of Digital Media in Norway, experimented with ChatGPT, she found that although the chatbot could converse in Norwegian, it had limited knowledge of Norwegian life and culture (Walker Rettberg, 2022). Similarly, AI-generated fiction set in Australian locations often lacks culturally or geographically specific references. A generic reference to ‘the Melbourne skyline’ does nothing to create a sense of place compared to the vivid rendering of familiar settings, shops and food in authentic Australian YA.
LLMs often lack a distinctive voice
Large language models often struggle to maintain a unique narrative voice and the writing can be bland and prescriptive. This is because LLMs are designed to select the most likely word in a sequence, the measure of which is called ‘perplexity’. However, to avoid boring prose, there needs to be an element of ‘burstiness’ in written expression, which is the unexpected or creative use of language. As one might expect, LLMs value predictability over burstiness, prioritising safety and correctness (Mukherjee, 2023). To compound the issue, LLMs are strictly trained to avoid profanity or potentially offensive terms, sometimes overcompensating and removing unlikely or interesting words. This can result in characterless yet correct prose.
Their preference for predictability, together with their lack of cultural nuance, means LLMs often struggle with slang, which is inevitably regionally distinct. Moreover, the filtering of datasets tends to result in the removal of non-American English dialects. Slang is important to cultural expression; it literally means ‘on the margins’, which points to its subversive qualities and culturally distinctive characteristics (Madison, 2023). Students might explore the use of informal language or Australian vernacular by comparing dialogue in Australian YA and AI-generated texts. The different style of humour might also be evident. One Australian author noted that Australian fiction is decidedly less ‘saccharine’ than US fiction.
LLMs revert to tropes
The safety mechanisms designed to prevent large language models from generating inappropriate content can also result in conservative plot choices. In a study of professional writers using chatbots as writing aides, one author observed that the chatbot ‘seemed very reluctant to generate people doing mean things’ (Ippolito et al., 2022). Yet literature explores the wide gamut of human experience, representing human frailties as well as strengths. Similarly, authors noted chatbots were predisposed to revert to tropes and archetypes (particularly from the fantasy genre) in their plot suggestions (Ibid). Such a tendency hinders creative progress and undermines authentic exploration of the human experience.
In her recent report on generative AI, Stefania Giannini, UNESCO's Assistant Director-General for Education, insisted that ‘language matters’ because it is ‘at the heart of identity and cultural diversity’ (Giannini, 2023). This is why it is imperative for students to be exposed to quality Australian texts that reflect their unique experiences of culture and place. Through the process of comparing passages from Australian YA novels with AI-generated equivalents, the limitations of generative AI can be made explicit to students while fostering a love of Australian stories that are about them and for them.
This project is supported by the Copyright Agency’s Cultural Fund.
References
- Levenson, M. (2023, February 23). Science Fiction Magazines Battle a Flood of Chatbot-Generated Stories. The New York Times. Retrieved January 31, 2024, from https://www.nytimes.com/2023/02/23/technology/clarkesworld-submissions-ai-sci-fi.html
- Hepworth, S. (2023, February 10). Who said it: An Australian MP or ChatGPT? The Guardian. Retrieved October 27, 2023, from https://www.theguardian.com/australia-news/2023/feb/11/who-said-it-an-australian-mp-or-chatgpt
- Caliskan, A. (2021, May 10). Detecting and mitigating bias in natural language processing. Brookings. Retrieved February 02, 2024 from https://protect-au.mimecast.com/s/YeDxC0YK7MCGgoxgUwkCT9
- Dodge, J., Marasovic, A., Ilharco, G., Groeneveld, D., Mitchell, M., & Gardner, M. (2021). Documenting Large Webtext Corpora: A Case Study on the Colossal Clean Crawled Corpus. Conference on Empirical Methods in Natural Language Processing.
- Walker Rettberg, J. (2022, December 06). ChatGPT is multilingual but monocultural, and it's learning your values. Jill/txt. Retrieved December 08, 2023 from https://jilltxt.net/right-now-chatgpt-is-multilingual-but-monocultural-but-its-learning-your-values/
- Mukherjee, S. (2023, June 15). The Significance of Perplexity in Evaluating LLMs and Generative AI. Medium. Retrieved November 09, 2023 from https://ramblersm.medium.com/the-significance-of-perplexity-in-evaluating-llms-and-generative-ai-62e290e791bc
- Madison, C. (2023, October 28). Why AI Doesn't Get Slang. The Atlantic. Retrieved February 02, 2024 from https://www.theatlantic.com/family/archive/2023/10/can-chatgpt-use-slang/675828/
- Ippolito, D., Yuan, A., Coenen, A., & Burnam, S. (2022). Creative Writing with an AI-Powered Writing Assistant: Perspectives from Professional Writers. arXiv [Cs.HC]. Retrieved from http://arxiv.org/abs/2211.05030
- Ibid
- Giannini S. (2023). Reflections on generative AI and the future of education. © UNESCO. Retrieved from https://unesdoc.unesco.org/ark:/48223/pf0000385877